
Description
With reference to data size of miniImagenet, we construct multiple few-shot datasets to analyze the performance differences from image complexity. We construct some datasets with different image complexities, which include the simple character image and the images with different number of objects, e.g., general object images and scene images. In addition to the above images, facing vertical fields, we establish some fine-grained image datasets including food and flower datasets. To avoid effect from the number of categories and images, we guarantee all few-shot datasets have the same number of category and image per category with reference to the number of categories and samples in each category in miniImagenet. Sorted by image complexity, the datasets mentioned are miniCharacter, miniImagenet, miniPlaces, miniFlower and miniFood.
Diverse dataset is used to investigate the impact of transferable capabilities learned from distributions of base categories. The distribution are described with instance diversity and category diversity under settings of relevant or irrelevant novel categories.
Structural dataset is used to analyze differences in performance on different datasets from characteristics of dataset structures and different few-shot learning methods. It contains five sub-datasets with different structures, including miniCharacter, miniImagenet, miniPlaces, miniFlower, miniFood.
Datasets
Diverse dataset. This dataset is sampled from ImageNet [1], including diverse categories and diverse instances. These categories and instances are used to form base categories and novel categories according to the tree structure in ImageNet. As illustrated in Fig XX, the root of the tree structure is divided into two branches. The first branch is continuously divided into the dog branch and the Living Thing Except Dog (LTED) branch. The second one is divided into the ARtifact INstrument (ARIN) branch and the Artifact Other Thing branch (AROT). The base categories (i.e., original base categories, additional base categories) and novel categories we use are sampled from the LTED, ARIN, and AROT branches.
[1] Russakovsky, Olga, et al. "Imagenet large scale visual recognition challenge." International journal of computer vision 115.3 (2015): 211-252.

Structural dataset. This dataset's sub-datasets are detailly described below.
- miniCharacter. We collect and establish a handwritten character dataset, called miniCharacter, which is generated and annotated by 15 volunteers. MiniCharacter includes various characters such as english letters, numbers, mathematical symbols. The total number of categories is 84, and each of category has 100 images. The 64, 20 categories are used as base, novel categories, respectively.
-
miniImagenet. MiniImagenet is firstly used by, which uses 64,20 categories as base, novel categories, respectively. And each category contains 600 images.
- miniPlaces. Sampled from Places365, we construct MiniPlaces, which uses 64, 20 categories as base, novel categories, respectively. And each category contains 600 images.
- miniFlower. Sampled from flower dataset provided from 2018 FGCVxFlower Classification Challenge, we construct MiniFlower, which uses 64, 20 categories as base, novel categories, respectively.
- miniFood. Sampled from Food101, we construct MiniFood,whichuses64,20categoriesasbase,novel categories, respectively. And each category contains 600 images.
Example images in Structural dataset

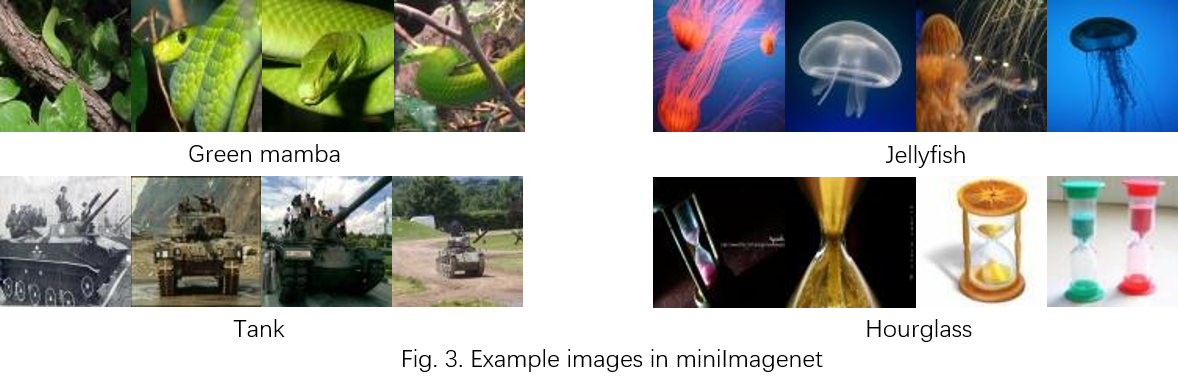
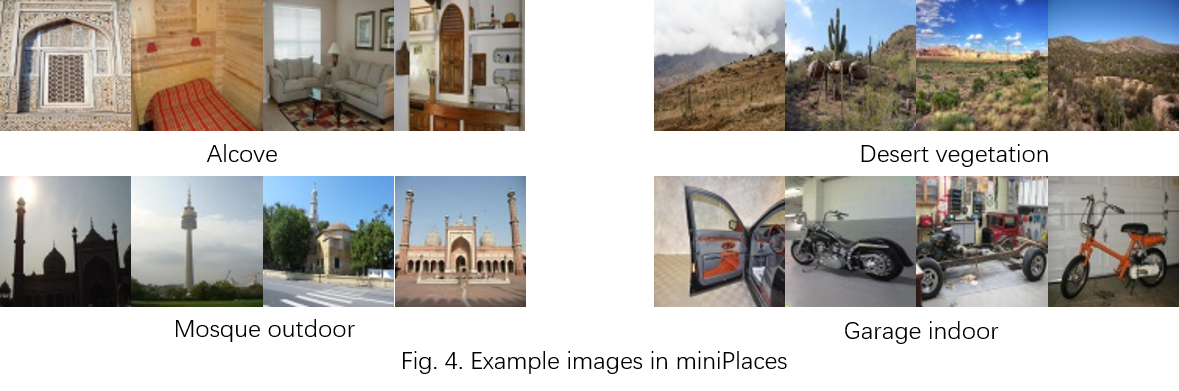
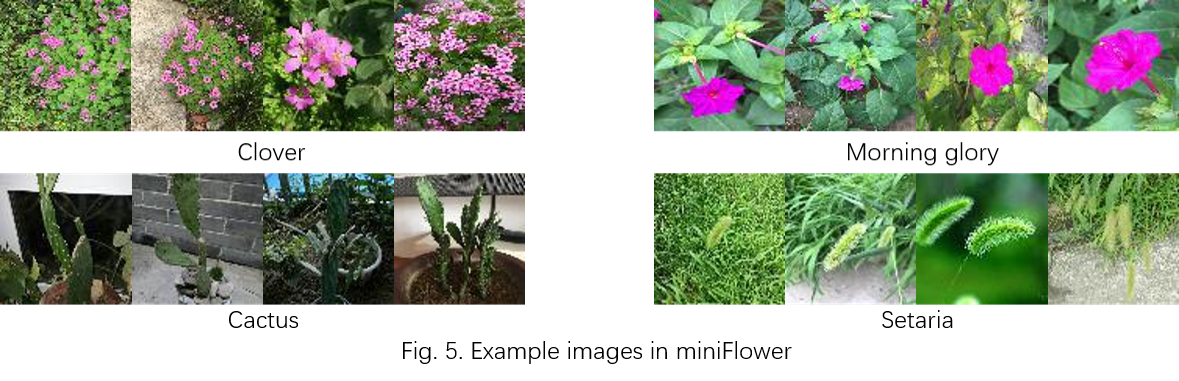

5-way 1-shot accuracy (%) on different datasets with ResNet-12
Datasets | Metric-based methods | Meta-based methods | ||||||
PN[2] | RN[3] | DSN[4] | DEMD[5] | MAML[6] | adaCNN[7] | Proto-MAML[8] | MetaOpt[9] | |
miniCharacter | 92.87 | 92.15 | 92.70 | 93.49 | 85.65 | 89.26 | 94.24 | 94.30 |
miniImagenet | 58.47 | 53.08 | 58.67 | 60.46 | 54.90 | 57.13 | 57.37 | 59.73 |
miniPlaces | 60.55 | 58.01 | 61.30 | 60.47 | 58.25 | 56.35 | 60.40 | 63.12 |
miniFlower | 55.63 | 55.36 | 55.26 | 61.62 | 54.15 | 58.10 | 55.13 | 58.53 |
miniFood | 51.35 | 49.29 | 51.58 | 54.76 | 49.44 | 52.15 | 50.63 | 52.30 |
[3] Sung, Flood, et al. "Learning to compare: Relation network for few-shot learning." Proceedings of the IEEE conference on computer vision and pattern recognition. 2018.
[4] Simon, Christian, et al. "Adaptive subspaces for few-shot learning." Proceedings of the IEEE/CVF Conference on Computer Vision and Pattern Recognition. 2020.
[5] Zhang, Chi, et al. "DeepEMD: Few-Shot Image Classification With Differentiable Earth Mover's Distance and Structured Classifiers." Proceedings of the IEEE/CVF Conference on Computer Vision and Pattern Recognition. 2020.
[6] Finn, Chelsea, Pieter Abbeel, and Sergey Levine. "Model-agnostic meta-learning for fast adaptation of deep networks." International Conference on Machine Learning. PMLR, 2017.
[7] Munkhdalai, Tsendsuren, et al. "Rapid adaptation with conditionally shifted neurons." International Conference on Machine Learning. PMLR, 2018.
[8] Triantafillou, Eleni, et al. "Meta-dataset: A dataset of datasets for learning to learn from few examples." arXiv preprint arXiv:1903.03096 (2019).
[9] Lee, Kwonjoon, et al. "Meta-learning with differentiable convex optimization." Proceedings of the IEEE/CVF Conference on Computer Vision and Pattern Recognition. 2019.